The Predictive Maintenance Maturity Journey
There is no real argument left to be had about the benefits of condition-based predictive maintenance over time-based preventive maintenance.
Most manufacturers who have invested in reliability by introducing machine health monitoring technology have quickly realized ROI and seen significant productivity gains as a result. Other manufacturers who still stick to preset maintenance schedules and conduct route-based monitoring continue to struggle with managing their overall maintenance spend and increasing Operational Equipment Effectiveness (OEE).
The Data Backs it up.
A 2022 Manufacturers’ Alliance report found:
Where many manufacturers stall out is after reaching a base level of predictive maintenance implementation. Most maintenance maturity models show predictive (or prescriptive) maintenance as the destination. At a high level, this is correct, but it leaves out half of the journey. There are many stages of maturity left to reach once you adopt a predictive or condition-based approach to maintenance.
This guide will shine light on that second half of the journey, walking you through the four pillars of predictive maintenance maturity and helping you accelerate the revenue gains that Predictive Maintenance (PdM) enables.
The First Half of the Journey
This is the advanced guide to predictive maintenance maturity, so we assume you know why so many smart manufacturers are motivated to adopt a condition-based approach to maintenance. Still, it’s useful to revisit the first half of the journey in order to better understand the second.
Manufacturers who have not yet started on their predictive maintenance journey still practice reactive maintenance. This approach—running machines to failure before they fix them—is an excellent strategy if you’re waiting to change a lightbulb. Otherwise, it results in a high number of unplanned downtime hours, shrinking margins, and stressed-out maintenance teams.
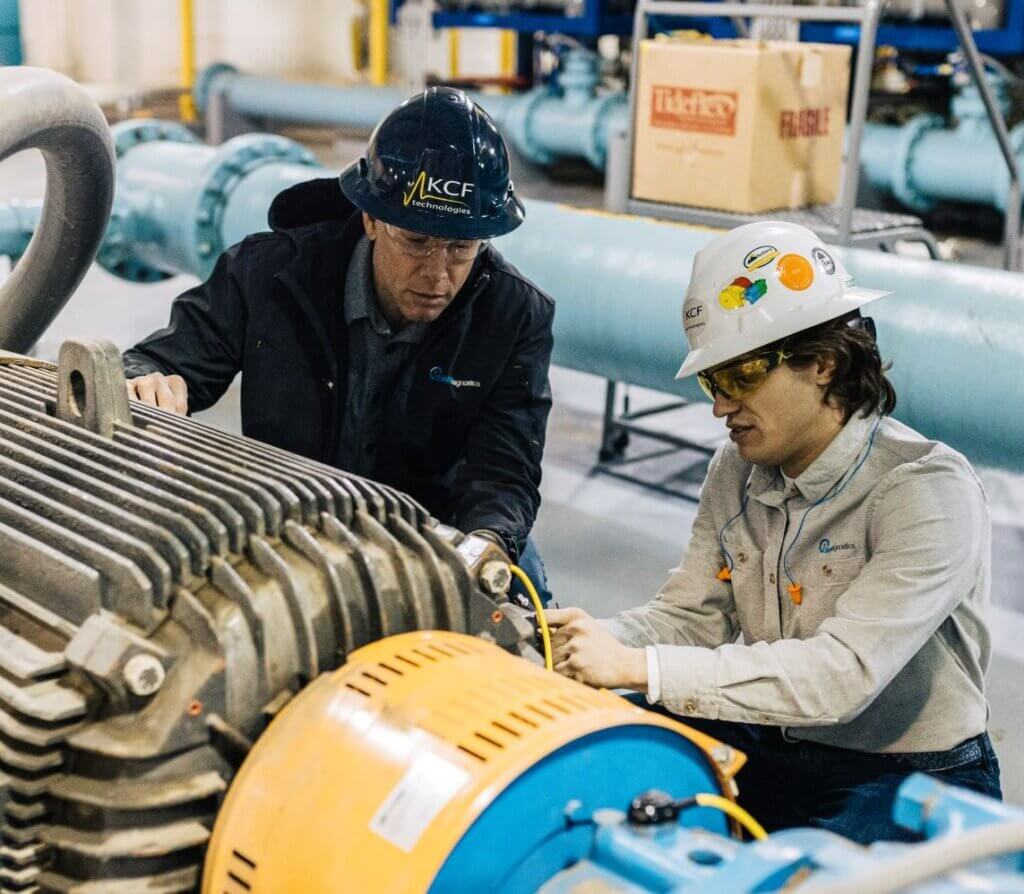
Practicing reactive maintenance means making a conscious choice to allocate most of your maintenance resources (and thus a high percentage of your total operating expenses) to reacting to problems. Reacting to machine health problems is often confused with solving machine health problems.
Preventing machine health problems— the goal of preventive maintenance—is also frequently confused with solving machine health problems. Preventive maintenance tends to be time-based or usage-based, meaning maintenance teams complete regularly scheduled tasks that are meant to keep assets in peak working condition. This approach is only successful some of the time and tends to be wasteful. Machines don’t break down at regular calendar intervals, and unnecessary Preventive Maintenance (PMs) don’t solve chronic asset health issues.
In this first half of the maintenance maturity journey, the most significant step is moving from time-based to condition-based maintenance. With the addition of continuous monitoring hardware, manufacturing maintenance teams can perform reliability tasks that are tied to the actual condition of an asset, eliminating wasteful PMs and reducing unplanned downtime. Condition monitoring enables predictive maintenance, helping manufacturers understand the correlation between the timing of maintenance actions and machine performance.
This is the basic journey to maintenance maturity. If you’re not here yet, you’re certainly not alone—many manufacturers still operate with a mix of run-to-failure and preventive maintenance strategies. Of course, many manufacturers also still struggle to stay competitive and improve their bottom line. In our experience, the two are often connected.
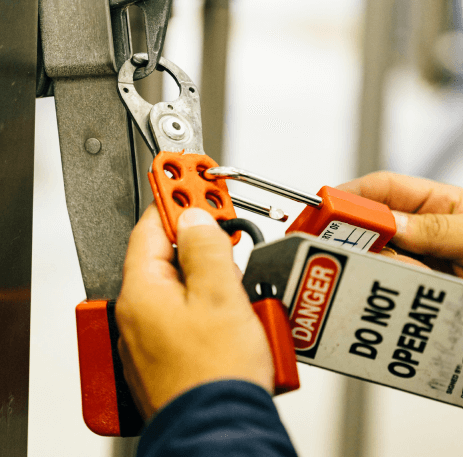
Unlocking the Second Half of the Journey
Using Industry 4.0 Technology
The rest of the maintenance maturity journey—the real focus of this guide—comes after the initial adoption of condition-based maintenance technology. Connected technology is the key to continuous reliability improvement and forms one of the four pillars of true predictive maintenance maturity.
With the right technology and expertise fueling their reliability efforts, manufacturers can achieve better decision-making intelligence and more efficient business models, leading to greater operating flexibility, lower overhead, and higher productivity. Modern machine health technology doesn’t just observe your assets; it reveals the behavior of machines under different operating conditions and enables accurate root cause analysis so you can permanently solve your asset health problems.
The Four Pillars of Predictive Maintenance Maturity
There are four pillars-or key areas of growth-for manufacturers who want to advance their predictive maintenance strategy.
1. Instrumentation
2. Integration
3. Insight Generation
4. Optimization
Making gains in each key area is not a strictly linear process, but the basics of each should be mastered before moving on to the next pillar. For example, manufacturers have to instrument their assets with the right condition monitoring hardware before tackling integration, and need integrated systems to begin work on insight generation.
Let’s take a deeper dive into each of the four pillars.
1. Instrumentation
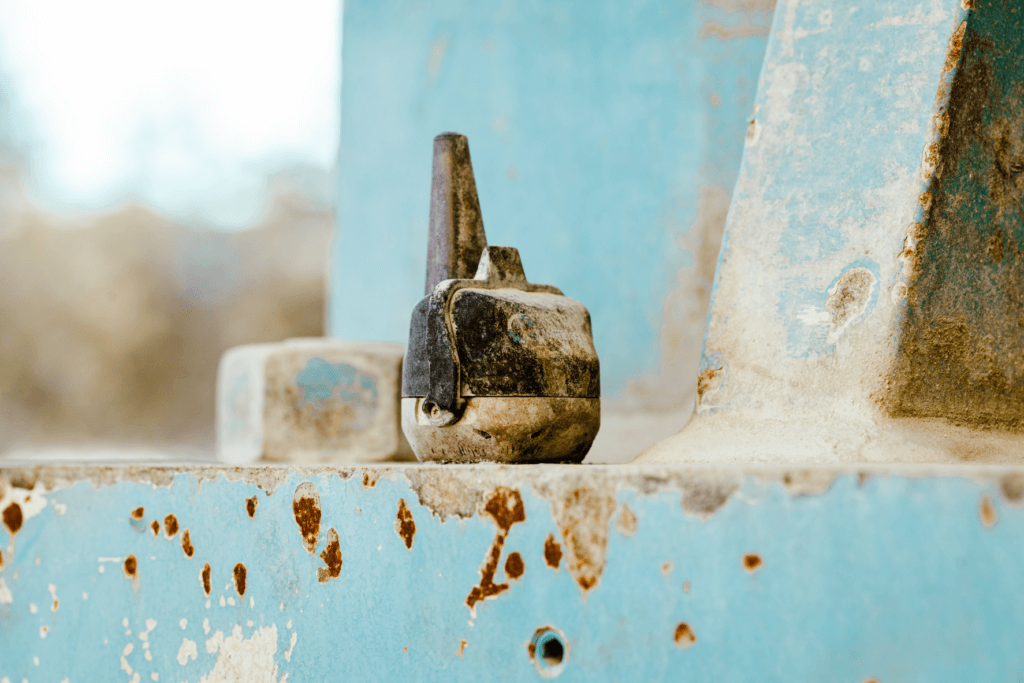
Starting with a limited amount of hardware as a pilot—for example, only instrumenting one asset class for remote monitoring—is an approach that many manufacturers take when adopting condition-based maintenance, and is a fine way to prove ROI before wider implementation. For this reason (among others), scalability is an important consideration when choosing a machine health monitoring hardware vendor.
Many vendors promote hardware flexibility as a benefit of their products, but “flexibility” is often “lack of support” in disguise. Of course, flexibility to monitor a range of machinery, including machinery that operates intermittently, is important. However, there is always an optimal way to instrument any given asset in order to catch issues that are unique to that asset. With flexibility sometimes comes lack of standardization. If you have two identical assets in two separate plants that are instrumented differently, the data you collect won’t help you identify common issues and implement best practices across your entire organization.
Look for scalability paired with in-house engineering and machine health expertise from your vendor to ensure a standardized rollout, along with help in interpreting the initial data you collect and realizing early wins. You should be able to eliminate catastrophic events with an initial physics-based rollout covering the most common rotodynamic assets, using preset algorithms to detect key fault signals. From here, you’ll establish machine Key Performance Indicators (KPIs) and operational baselines to determine the right alarm thresholds.
As manufacturers progress with instrumentation maturity, additional sensor types can be introduced, including sensors that can accurately monitor machinery that operates intermittently. Assets with repeated loading and unloading cycles are highly susceptible to vibrational damage, but traditional vibration monitoring can’t reliably catch failures for these machines.
Network reliability is also a critical factor in instrumentation maturity. Relying completely on WiFi or cellular signals means inevitably missing data points that could point to imminent failure. A sophisticated instrumentation strategy includes a reliable mesh network that provides always-on coverage to even the most remote areas of your plants, with data backup infrastructure to ensure you don’t miss a beat if your network fails.
The instrumentation pillar includes the software that supports your hardware deployment. Your data is only useful if it can be routed, interpreted, and visualized in a way that is actionable for your team, surfacing process anomalies and impending failures right away. Scalability is important here, too— you should be able to benefit from actionable data on day one, but have the ability to deploy advanced custom dashboarding, role-based alerting, and integrations.
2. Integration
Every modern manufacturing facility relies on a number of software platforms to keep their operations running smoothly. Each has an important role to play, but disconnection between platforms can lead to missed insights and wasted resources.
Machine health monitoring software is especially susceptible to operational efficiency misses caused by lack of integration. Maintenance actions are usually organized, prioritized, and recorded via CMMS, with machine health software operating in a separate silo. Information about operator Standard Operating Procedures (SOPs) and setpoints may not be connected to either system—often, they’re just considered institutional knowledge and can vary dramatically from line to line or operator to operator.
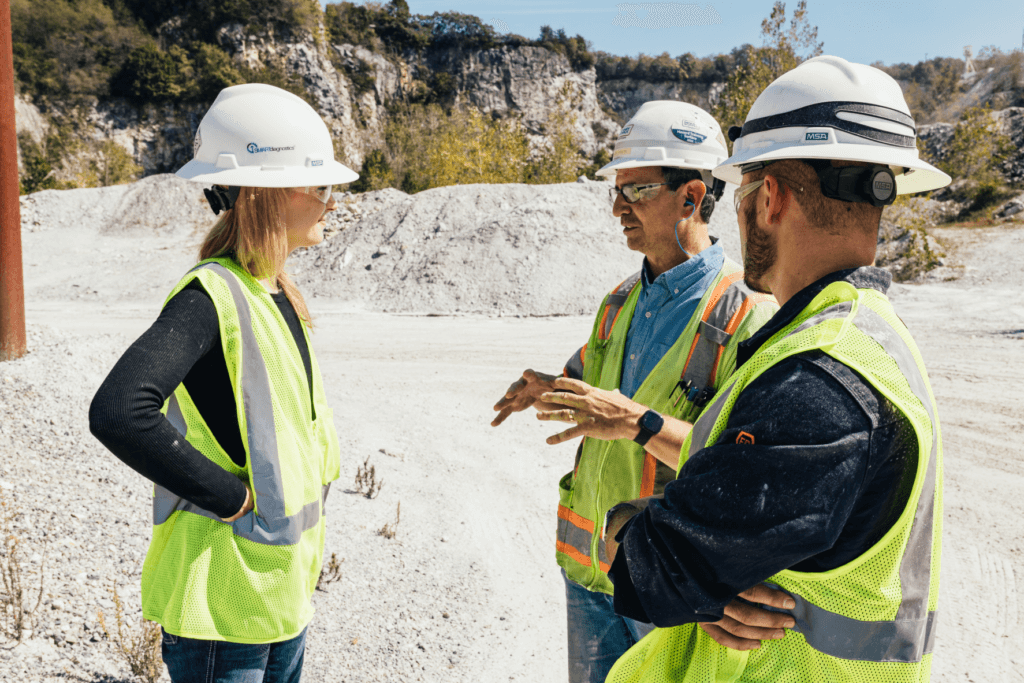
Integration maturity includes building connectivity between your machine health monitoring software, a diagnostics engine to enable prescriptive maintenance, your CMMS for closed-loop maintenance workflows, and other critical data platforms (for example, your ERP for spare parts workflows).
If these platforms stay siloed, manufacturers can miss out on some of the most valuable benefits offered by advanced predictive maintenance strategies, like maintenance prioritization and validation. More than half of preventive maintenance activities have a neutral or even negative effect on machine health, but it’s impossible to know which half without a means to record and measure the effect of maintenance activities within the context of your machine health data. An asset monitoring platform that is disconnected from your CMMS can’t easily surface this kind of critical information, nor can it provide criticality analysis or prioritization.
Large manufacturers (or highly specialized smaller organizations) will often have custom integration and/or data security requirements for their enterprise data architecture. Your vendor should be able to handle those with an in-house IT and engineering team capable of ensuring all systems are strategically connected and pulling the right data (and only the right data) to fuel actionable machine health insights.
For quick gains in this area of predictive maintenance maturity, look for a solution that offers standard process and CMMS integrations versus one that requires a custom, hands-on setup every time you integrate a new system.
3. Insight Generation
Manufacturing leaders know that the promise of Industry 4.0 isn’t a fully automated smart factory requiring minimal human inputs. People have always been, and will continue to be, our most valuable manufacturing resource. Sophisticated manufacturers invest in generating insights so that their people are always empowered to take the most informed, efficient, resultsfocused actions on the shopfloor.
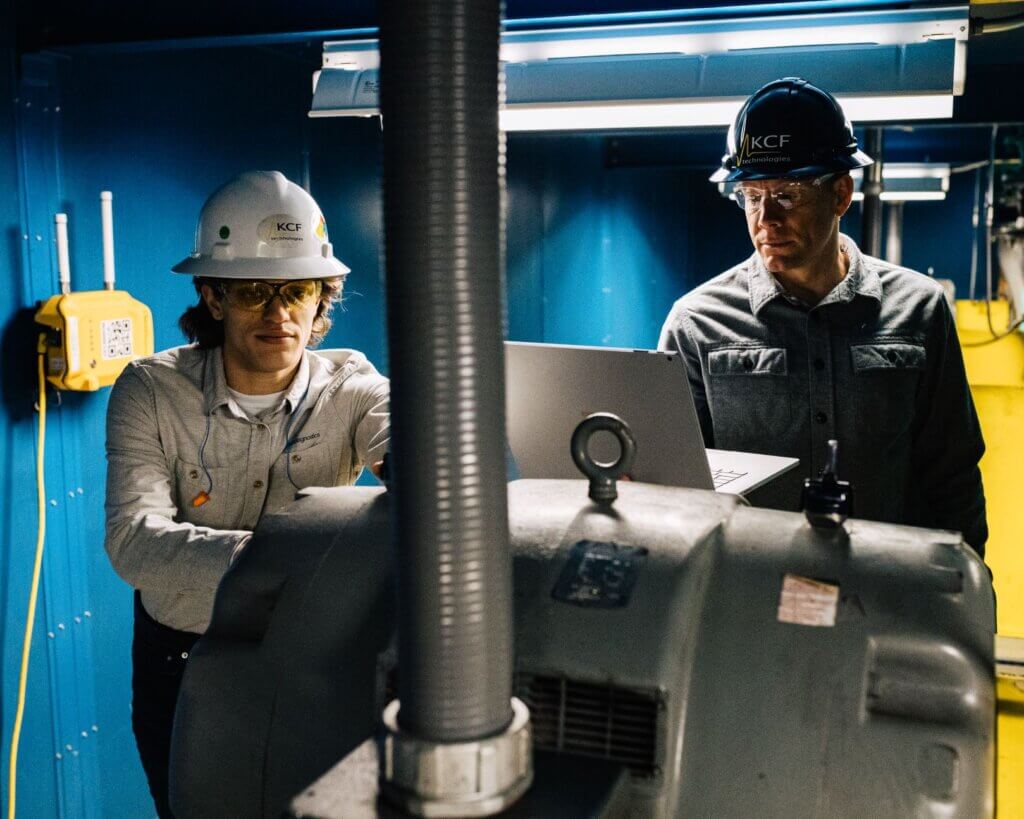
“Entry-level” insight generation on your journey to predictive maintenance maturity starts with automated alarming (tailored to your machines’ baselines).
Advancements in insight generation are only unlocked when you’ve reached a level of instrumentation maturity that gives you the ability to identify anomalies and take action on them. If your remote monitoring equipment delivers raw data that only a certified vibration analyst can read, insight delivery suffers from serious lag, costing you productivity and bottom-line revenue.
Achieving maturity in the area of insight generation begins with choosing a platform that is capable of getting the right information to the right people with minimal friction along the way. Easy-to-understand visualizations (for example, asset scorecards that make it clear which of your machines need maintenance attention and the recommended order of priority for each) are key.
Insight generation shouldn’t be a black box. Although pre-built algorithms for common asset classes are a must-have, extremely precise issue classification can only be achieved by incorporating shop floor and subject matter expert feedback. The actual day-to-day and hour-to-hour operating conditions within your plant are a critical factor in creating automated insights and delivering them to the people who can make a difference.
Proving that people are the most critical asset in any manufacturing environment, even with fully automated insight delivery, human intervention is necessary at every step to take machine health insights and turn them into increased operational efficiency. Operators, managers, and reliability professionals have to work together to improve operating conditions based on the recommendations provided by a sophisticated machine health platform. Cultural change is key, but can be difficult—the right machine health optimization partner can help you with change management that is tailored to your unique environment.
4. Optimization
The final pillar of predictive maintenance maturity represents an area of nearly infinite growth potential. Manufacturers who invest in the right instrumentation, integration, and insight generation technology and expertise will continuously find new ways to optimize their operations.
In the first phase of the predictive maintenance maturity journey, manufacturing teams move from constantly putting out fires to being able to predict and avoid imminent failure. In this second phase, manufacturers can learn to engineer failure out of their processes entirely.
With the right data collected by the right hardware, plant conditions—including variables introduced by different operators, different products, and different processes— can be fully understood. Manufacturers can then take advantage of machine learning and AI to determine the best way to run a machine. This looks like ongoing optimization on the fly, based on current conditions, to maximize productivity and fully eliminate unplanned downtime.
Beyond that, a smart machine health optimization platform can help reliability teams reduce planned downtime by gathering information about previous maintenance actions and helping teams determine which of them are useful and when. Robust historical machine health data can tell you how long a machine can run before truly needing planned maintenance, helping you schedule downtime around critical production times.
A production environment that has zero waste, zero safety incidents, zero unplanned downtime, and minimally disruptive planned downtime is within reach for every manufacturer willing to make the journey.
Continuing Your Predictive Maintenance Journey
Achieving an advanced predictive maintenance strategy requires a number of critical components that need to be tightly integrated. Custom integration, data cleansing, and working out interoperability can take years to get right. The first step toward success should be choosing a full-stack solution, dramatically decreasing time-to-value.
The tools to drive operational change are simply a means to an end. A shift in worker practices is required, and needs to be prepared for. If your maintenance team keeps over-greasing bearings even though your machine health software flags the asset and recommends that the PM was ineffective, the bearing will continue to fail prematurely.
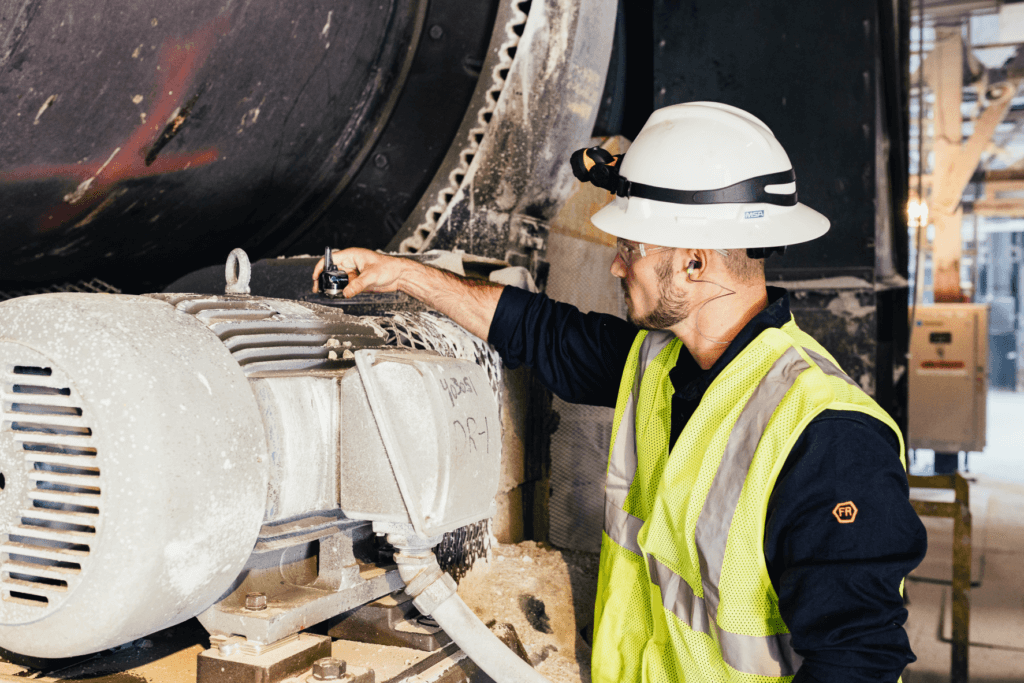
The value promised by predictive maintenance maturity will only be achieved when leaders, managers, maintenance professionals, and operators change the way they do their job on a day-to-day basis. Too often, initial excitement for a new solution or method is replaced with complacency and, ultimately, a return to legacy practices.
Practical adoption of your maturing predictive maintenance strategy needs to be supported by a growth-oriented partner that offers education and training services. They should also offer industry-specific expertise, helping you properly instrument your machinery and provide advanced data analysis.
The farther along in your predictive maintenance maturity journey you get, the more ROI you will be able to see from your investments, and the more you will differentiate yourself from your competitors.
KCF’s Offerings
for the Four Pillars of Maintenance Maturity
KCF exists to elevate people and solve Industry 4.0 problems. We envision a world where our customers have zero injuries, zero waste, and zero asset failures. We effectively connect cutting-edge technologies with real-world solutions that improve industrial facilities and the communities they serve for a sustainable future.
OUR MISSION
Instrumentation
KCF OFFERS: Reliable data transmission using DARTwireless protocol; Extended battery life for ease of use and installation; Comprehensive coverage of all assets—whether common rotating equipment or complicated, intermittent assets.
Integration
KCF OFFERS: Standard integrations with plant processes data and CMMS data.
Insight Generation
KCF OFFERS: Analytics powered by 289,364 machine health hours; Built on a foundation of world class vibration knowledge, first pioneered by co-founder Gary Koopman, founder of the Center for Acoustics and Vibration; Dedicated team of engineers and vibe analysts to validate callouts and act as an extension of your team.
Optimization
KCF OFFERS: Integrations for full visibility; High frequency data for advanced root cause analysis; In-software tools for tracking maintenance effectiveness and informing ideal machine set points and maintenance practices; Team of SENTRY engineers to support and guide your team on their PdM journey.
Smarter Operations Start Here
BOOK YOUR SMARTdiagnostics DEMO
Our mission is to transform industry by solving critical machine health problems permanently. We develop smart, gritty solutions to tackle your toughest manufacturing asset challenges.
Are machine health challenges holding you back from gaining a competitive advantage and reaching your full revenue potential? We can help.