A Better Approach to Asset Health
Traditional approaches to managing machine health are no longer enough to get and stay competitive in today’s manufacturing landscape. Machine health optimization represents a new way of thinking and operating that can unlock the revenue-generating potential of your business and provide 10x ROI on your machine health investments.
This page contains a roadmap to guide you on your machine health optimization journey. First, you need to understand why the path you’re following today may be creating the suboptimal operating conditions that are holding you back.
There is a better way to manage the health of your assets, your plant, and your business as a whole.
The biggest challenge to overcome as you move toward machine health optimization is rejecting the way that things have always been done. If you perform an honest assessment, those traditional approaches to machine health management have likely resulted in:
- “Saving the day” but not addressing the root cause of equipment failure
- Maintenance being performed incorrectly
- Working reactively after a problem has occurred
- Data analysis that is out of touch with the day-to-day realities in your plant
- Operators not knowing how their set points impact equipment life
- Institutional knowledge driving maintenance, which becomes more and more dangerous as maintenance gurus move on or retire
- Inconsistent practices from plant to plant, or even between production divisions in the same plant
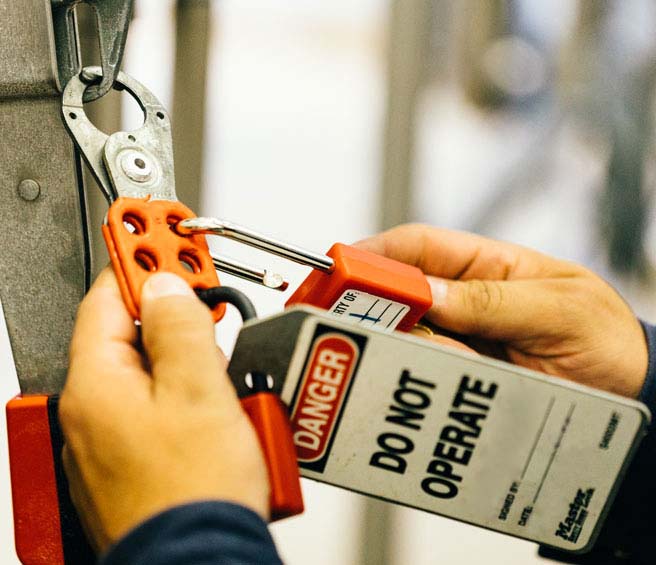
Reactive maintenance is an excellent strategy… if you’re waiting to change a lightbulb.
Over the past several decades, the conventional approach to machine health management has evolved beyond simple reactive maintenance — waiting for failure to happen before you do something about it. Reactive maintenance is an excellent strategy if you’re waiting to change a lightbulb, but much less so when you’re dealing with complex manufacturing equipment and the costly effects of unplanned downtime.
Most manufacturers today practice either preventive maintenance, predictive maintenance, or a combination of both. Neither approach achieves true optimization of your plant assets, but each is a good starting point.
Preventive Maintenance
Preventive maintenance (PM) was the first iteration of a better approach to managing machine health and is still widely practiced today. The driving theory behind PM is that machines are healthy when they are first commissioned and run in, and then wear out in a fairly predictable fashion over time. To avoid failure, maintenance is scheduled in regular intervals — usually based on hours of uptime or calendar days — before failure is predicted to occur.
This approach works…in theory.
If you know roughly how long a machine and its components are expected to last, you should be able to intervene before excessive wear occurs and avoid failure indefinitely. But anyone who has practiced PM knows that this is simply not the case; failure does still happen, and regularly. This makes preventive maintenance expensive as a rule. Service is often performed when it’s not needed, and approximately 80% of PM activities have a neutral or even negative effect on machine health.
Failure Is Almost Always Random
Any number of factors can lead to equipment failure — the products on your lines change, scheduled maintenance is performed incorrectly, or someone drops a tool on a machine. In this case, machine health degradation doesn’t follow a straight slope down to failure. It could even look like a straight line, with failure being equally likely at all times.
Decades of research show that random failure models are more accurate than predictable wear-out models more than 80% of the time. Only a small minority of equipment exhibits predictable wear-out behavior.
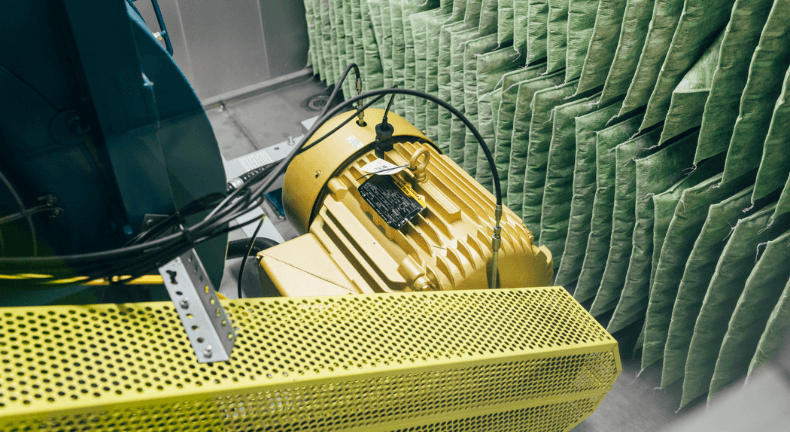
Predictive Maintenance
Once manufacturers figured out that so-called random failures were at least as likely to occur as predictable failures, predictive maintenance (PdM) became the next evolution of machine health management. The premise of predictive maintenance is that machine health conditions can change rapidly and degradation is not always predictable without additional data, so machines must be monitored to determine whether they’re healthy or about to fail.
Machine health monitoring, or condition monitoring, gathers data about machine performance via sensors, usually measuring overall vibration. If an abnormal vibration signature is detected, the maintenance team knows that something is wrong and can investigate the asset. When people think about PdM, they’re usually focused on what kinds of technology can detect potential failures as early as possible, so maintenance can be performed in between detection and failure in a safe, planned way.
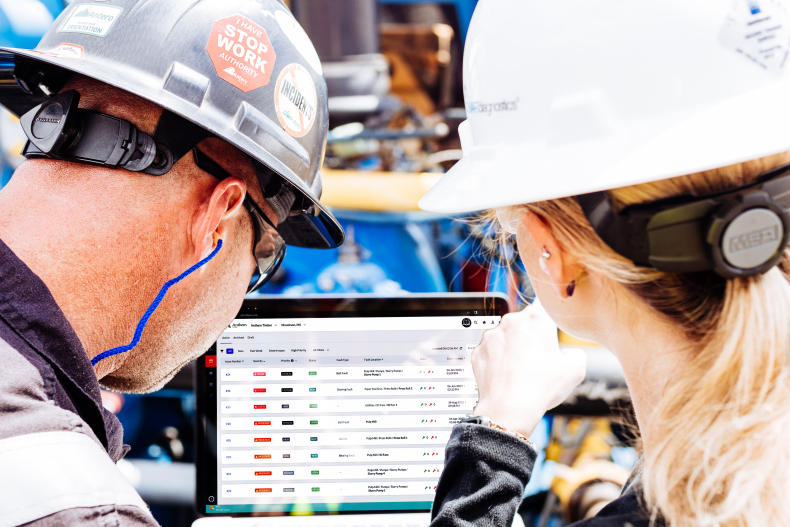
Route-based condition monitoring is still common, so maintenance leads are working with a rough sketch of machine health drawn with only a few data points as reference material. If route-based data collection only happens monthly or quarterly, false trends can emerge and steer maintenance teams in the wrong direction.
One of the major problems with PdM is that it often relies on bad or incomplete data.
Measuring Machine Health by OEE
Regardless of a manufacturer’s approach to maintenance, most businesses use overall equipment effectiveness (OEE) as the primary benchmark for asset health and, as an extension, overall plant health.
This is not entirely wrong, but it’s also not entirely right.
OEE measures mechanical performance, but in some ways it is a self-limiting measure. OEE generally tells you how well your plant can perform under its current operating conditions. Gains can be made in the areas of asset uptime, performance, and production quality, but often a gain in one of these areas is achieved at the expense of another.
This doesn’t have to be the case — OEE can be genuinely and effectively improved beyond your plant’s current capabilities, and can support the improvement of other operational KPIs — but traditional approaches to machine health management won’t let your plant reach its full potential or set you apart from your competition.
Focusing solely on OEE also ignores other critical operational factors.
What’s Wrong With Traditional Approaches to Machine Health Management?
Preventive maintenance is based on the idea that machines wear out predictably over long periods of time. Predictive maintenance argues that machines can fail at any time, so we must be constantly vigilant.
Both theories are wrong.
Equipment failure is not consistently predictable, but the events that lead to failure are not random — they’re systemic. And because they’re systemic, they’re almost completely avoidable.
Compared to reactive maintenance…
- Preventive maintenance costs 28% less
- Predictive maintenance costs 50% less
The Journey to Optimizing Machine Health
Manufacturers are feeling pressure to get on board with Industry 4.0 and Industrial Internet of Things (IIoT) technology, but the practical implementation of this tech has yet to happen for most. A significant number of challenges stand in the way; fitting together overlapping or competing solutions, negotiating the balance between plant and corporate initiatives, reconciling tried-and-true processes with innovative technologies, and figuring out the timing and expense of upgrading existing infrastructure.
Smarter manufacturing starts with machine health optimization.
Machine Health Optimization: Where to Start?
Manufacturers who are ready to embrace digital transformation should start with an initiative that delivers the highest likelihood of performance improvement and near-term ROI. This makes machine health a top candidate due to its impact on business-wide KPIs.
Remember: equipment failure is neither predictable nor random. In the vast majority of cases, it’s systemic — caused by avoidable conditions and behaviors that are driving unnecessary machine damage and suboptimal performance in your plant. Installation errors, inconsistent operator inputs, and improperly conducted maintenance are all things you can control if you can track them, identify them, and eliminate them.
The journey to machine health optimization includes some important milestones:
- Collecting critical data
- Solving early problems via correlation
- Introducing predictive analysis
- Establishing standard operating procedures for optimal performance
- Using machine learning to accelerate performance
- Automating away manual intervention
Collecting Data for Machine Health Optimization
Vibration monitoring is often where PdM data collection starts and ends, but it’s just the beginning of an optimization-focused approach to machine health. Somehow, you have to measure the health of your machines, and sensors are the ideal way to do that.
When people talk about condition monitoring, they’re often still thinking about route-based data collection. We used to pay people to walk around collecting data from vibration sensors on a monthly basis. Thanks to advances in technology, it now makes much more sense to instrument machines with low-cost wireless sensors that can stream data continuously to a centralized location.
A distributed continuous asset health monitoring system that provides hundreds of data points per hour is critical to being able to spot systemic damage-causing conditions. The type of data points it provides is also a key consideration. It’s an excellent idea to monitor single values like peak acceleration, overall vibration, and temperature, but single-value trending will only show that there is a problem without providing insight into what that problem might be.
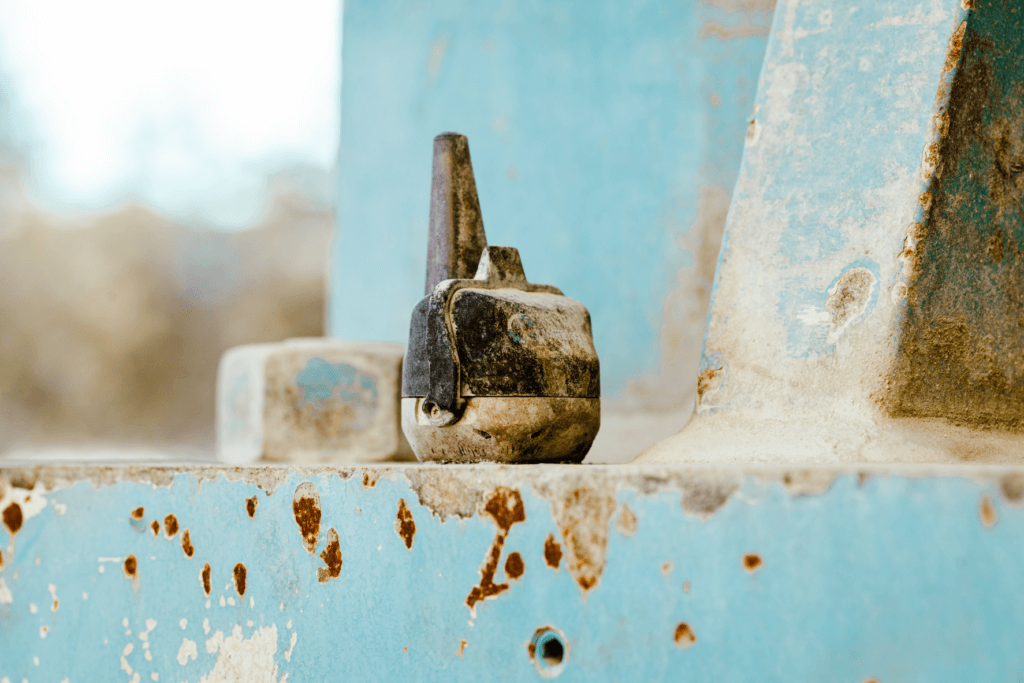
You might throw a lot of time and money at a single machine before you can identify the root cause of a problem.
There is more data out there. SMARTdiagnostics sensors send a time waveform, not a peak or an average. Then, the software shows that data in a frequency spectrum. Different kinds of mechanical faults look completely different in the frequency spectrum, but would look almost identical in the overall vibration chart.
As the field has matured, many other tools are now being used to comprehensively characterize machine health. This extends to not just simple rotating machines but many other types of equipment — ranging from intermittent running equipment like converting lines in packaging plants, to robots and lifts in automotive assembly.
Some manufacturers have cobbled together various sensor systems to try to gain a better understanding of overall machine health, but the best approach is one that leverages a common network infrastructure to connect all sensors under one umbrella; dramatically simplifying sensor and network maintenance, overhead, and management of the data.
Data collection using the right technology is an important first step, but sensors and data don’t push an actionable plan to the surface by themselves.
Solving Problems with Machine Health Optimization
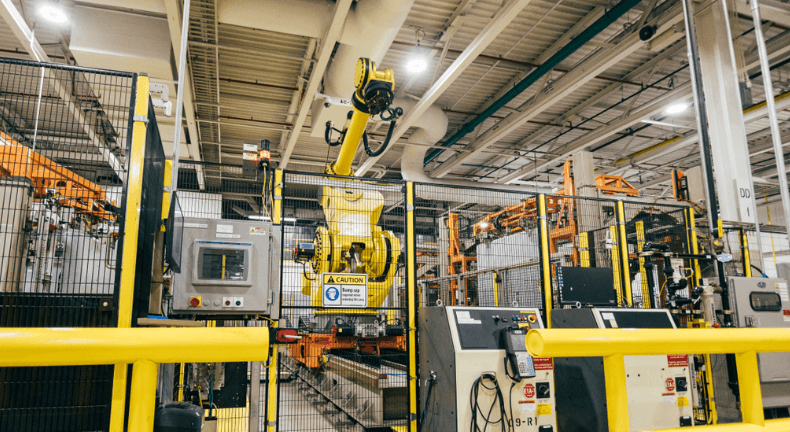
In order to create an action plan to optimize machine health, the data collected from your smart sensor network needs to be correlated with data about plant conditions and the people who interact with your equipment. By doing this, we learn which conditions lead to damage and which machine components are contributing. Then, we can engineer those damaging conditions out of the process. This leads to extended machine life, greater uptime, lower costs, and better energy efficiency.
This is the point where other machine health solutions providers might start talking about applying artificial intelligence to asset problems. AI is exciting and full of possibilities, but in the early days, it’s much more practical to capture knowledge from plant employees and adapt it into logic and digital workflows.
Many manufacturers think it could take weeks, months, or even years of monitoring to establish a useful baseline. Using SMARTdiagnostics sensor technology, we can collect thousands of data points within hours and get to work. Identifying failures gets easy very quickly, and catching failures equals quick ROI and a success story that everyone can rally around.
Early machine health optimizations wins are possible.
When you start putting sensors on every piece of important equipment, the resulting data avalanche can get overwhelming very quickly. It is not unusual to have tens of thousands of indicators in a single plant. For this kind of system to scale, the first step is auto-alarming, so the correct people can be notified when something is out of spec.
When you reach this point, congratulations — you’ve successfully started using an IIoT system to facilitate the goals of a predictive maintenance program. The journey to machine health optimization does not stop there. We can do much more to get even smarter.
Predictive Analysis and Machine Health
Implementing predictive analysis tools is where forward-thinking manufacturers begin to move past traditional PdM and toward true machine health optimization.
Machine health data has been collected and studied for decades, so many phenomena are already well-understood. We also have trillions of our own data points on tens of thousands of assets to supplement this global knowledge. With all of this information, we’ve built algorithms that can look at data coming in from a machine, compare it to thousands of known fault signatures, and quickly predict which fault conditions might be present in that machine.
At this stage in the journey, one of the primary goals is to deepen knowledge of your equipment’s health by understanding the physics of each machine and providing root cause analysis. For example, you may learn how changing pressure at a pump inlet influences other variables like vibration and temperature. Modeling and optimization at this stage are focused on finding the operating conditions that consume the least energy and minimize machine and component wear while still meeting production targets.
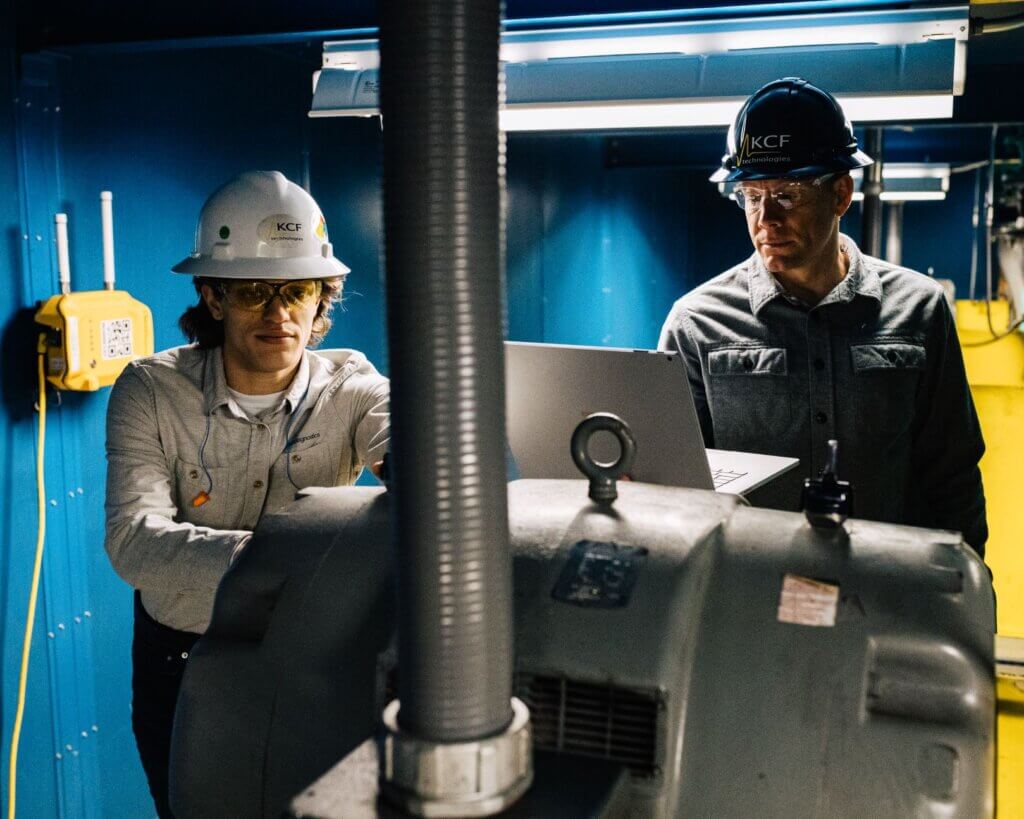
Optimizing for Machine Health
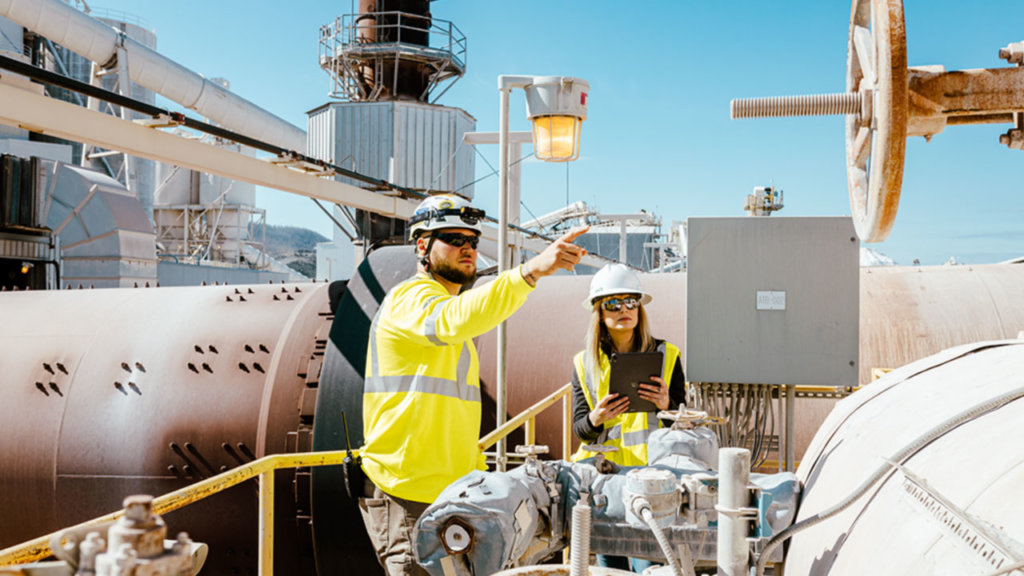
The final stages of the machine health optimization journey are what will drive the most value from your investments and solidly position you ahead of your competitors. Once damaging conditions have been identified and ideal operating conditions have been found, the next step is putting SOPs in place to ensure machines are consistently running in the best possible manner. This involves a number of factors that aren’t just OEE: machine health, energy costs, downtime costs, quality, labor, and so on. The definition of success includes both mechanical and business factors.
What actions will make the company the most profitable?
Once plant conditions are fully understood, we can use machine learning to apply advanced computational methods to determine the best way to run a machine. This looks like ongoing optimization on the fly — based on current conditions — in order to maximize value.
The final step is automation; removing as much of the human element from operational adjustments as possible. When this is fully and accurately implemented, the smart algorithms produced by the machine learning process can directly control your equipment, e.g. adjusting the speed of multiple parallel pumps in a system on-the-fly to minimize overall energy consumption, maximize output, or minimize machine damage.
The Impact of Machine Health Optimization
Depending on your approach to maintenance today, your plant could save as much as 67% of machine health costs by adopting an optimization-focused approach to machine health management.
The farther along in your machine health optimization journey you get, the more ROI you see from your IIoT investment, and the more you differentiate yourself from your competitors.
Through the convergence of people and technology, we can solve asset problems permanently and transform industry.
KCF partners typically see 10X ROI from their machine health optimization investments.
How to Get Started on your Machine Health Optimization Journey
An effective machine health optimization solution has a number of critical components. These components need to be tightly integrated, so a full-stack solution is recommended to support system performance, ease of use, and time-to-value. Custom integration, data cleansing, and working out interoperability can take years to get right. Full stack solutions dramatically decrease time-to-value.
To get started, you’ll need:
- An IOT platform that includes a range of sensors, enables connections to existing PLCs, and provides application-specific solutions.
- Advanced analytics for strategic asset management. At a glance, you should be able to learn the net present cost of a given operating state.
- Enterprise software that provides automated diagnostics and real-time machine health status. It should offer role-based customization, eliminating the need for multiple analysis solutions.
- An implementation partner that can offer a range of services, including training and education to help overcome the number one reason that Industry 4.0 implementations fail: lack of adoption.
KCF exists to elevate people and solve Industry 4.0 problems. We envision a world where our customers have zero injuries, zero waste, and zero asset failures. We effectively connect cutting-edge technologies with real-world solutions that improve industrial facilities and the communities they serve for a sustainable future.
“KCF doesn’t just sell a product and walk away. They work with you to monitor your machinery.”
– Reliability Manager
Contact Us
The journey to true machine health starts with a conversation. Contact us today to learn more about eliminating the root cause of your asset failures.