What Companies Often Get Wrong with AI in Manufacturing
January 28, 2022 | Artificial Intelligence, Machine Learning, Predictive Maintenance
by Jeremy Frank and Jacob Loverich
TOO LATE
While some companies are aggressively adopting AI, most are dabbling with it and planning to pursue a “fast-follower” approach—a strategy where companies wait for the technology and market to mature before pursuing it. This strategy has worked for some technology adoption in the past, but as noted in an AI focused special issue of the Harvard Business Review (HBR), it’s the wrong approach for AI. This is because AI is already sufficiently mature to make large improvement in business operations, and AI takes time to deploy during which job roles are adapted, and training data sets built. Therefore, the impact of AI will be latent and overlooked by late adopters, while early adopters will benefit from a combination of revenue growth, reductions in operating cost, and improved employee productivity, creating a winner takes all scenario. By the time the late adopters finish their AI journey, they will already have lost considerable market share.
Although most companies in the industrial and manufacturing sectors are working hard to incorporate digital technologies, and, in some instances, have genuinely transformed the way they operate, the AI component, is often lagging. With slim profit margins and a focus on incremental change through lean practices, it’s difficult for manufactures to truly reenvision their business and commit to applying AI at scale. Vikram and Mahidhar rightly point out in the HBR that even applying AI to a laundry list of applications won’t drive consequential change unless the business operations are reenvisioned, enabling workers to pivot in their roles in collaboration with AI.
“By the time the late adopters finish their AI journey, they will already have lost considerable market share.”
However, a complete AI-centric make over is enormously complicated involving too many moving parts, stakeholders, and projects. The right approach is to identify a crucial slice or domain within a company and rethink every aspect of that domain. Then build and scale a solution across the company within that domain. This requires going deep on a particular focus area and getting the full AI stack right from the training data to the algorithm implementations, human-AI interfaces, and job-upskilling.
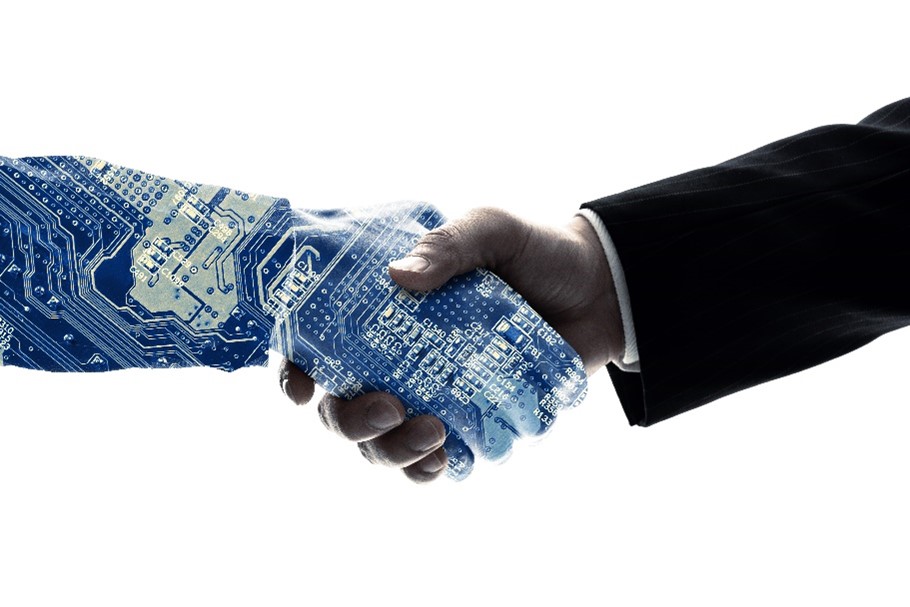
A domain that stands out in the manufacturing sector is machine health. Addressing pervasive machine health problems represents the single greatest opportunity to improve Overall Equipment Effectiveness (OEE). OEE is influenced by many factors, however, machine health and its relationship to maintenance and process operations holds the key to improving equipment availability through downtime avoidance, reducing defects, and increasing process rate, which are the key inputs to OEE.
For some early adopters, AI is already playing an essential role in the machine health domain by automating health diagnosis and maintenance recommendations. In some cases, AI is even going beyond diagnosis to automate process optimization based on the health of machines. For example, in hydraulic fracturing, KCF Technologies is using AI to control fleets of assets based on their health status. In addition to avoiding unexpected catastrophic failures, non-productive pump time during startup and shutdown was reduced by 40%. In another instance, KCF Technologies showed that AI models will predict failures 8 weeks earlier than could be achieved through human-driven analysis of the machine vibration signatures. A key aspect of both examples is that AI not only directly improved operations and the bottom line, but it also enabled workers to spend less time performing tedious repetitive tasks and more time performing higher value work like root cause analysis and strategic planning.
TOO LITTLE
Picking the right domain and experimenting with AI is a logical first step, however, companies must quickly move to the scaling stage. Again, AI will have little impact until sufficient training data is accumulated, and workers have adapted to a new way of operating with AI, both of which require scale. Tim Fountaine and Brian McCarthy wrote in the HBR four steps required to deploy AI at scale.
Step One: Set the Strategy
The key to set the AI strategy is picking the right scope. The scope should be large enough to significantly improve the business bottom line, address a systemic problem, be owned by single champion responsible for the entire value chain, and be reusable across the entire business not just in one application or plant. In the machine health domain, scope should include early fault detection, root cause analysis, and maintenance validation.
Step Two: Structure the Team
While data scientists are often thought of as the key role behind AI, in fact, quite to the contrary, the most important team members are domain experts. Domain experts like equipment reliability engineers work together with data scientists and organizational change leaders to shape a solution that leverages the right training data and can be practically applied.
Step Three: Reimagining Business as Usual
To realize consequential benefit of AI, businesses need to not just derive new insights from AI or automate a process, rather, new ways of operating the business will need to be reimagined. To explore this, work backwards from the end outcome that you are trying to achieve. For example, in the case of machine health, start with the root causes of unplanned downtime, waste, and safety incidents. By working backwards, poorly performed maintenance and adverse process behavior will be uncovered as the key factors that degraded machine health. AI’s role in addressing these chronic problems can then be envisioned.
Step Four: Adapt for Organizational and Technological Change
Upskilling the workforce, shifting responsibilities, and adjusting workflows are essential for AI to take hold. For example, dangerous equipment inspections need to be replaced with wireless sensors and the reliability engineers who were formerly conducting vibration monitoring need to interface with AI driven machine health analysis. Instead of interpreting complex datasets, the reliability engineer will receive automated alarms and use AI to learn how to permanently solve problems that arise.
TOO FOCUSED ON AI, NOT ENOUGH FOCUS ON ORGANIZATIONAL CHANGE
Its straightforward to envision AI’s inevitable application to the manufacturing sector, and more specifically why and how AI will bring tangible business benefit. AI deployment can also be readily achieved by partnering with companies that deliver products tailored for the domain you are tackling. However, the biggest hurtle to adopting AI is achieving organizational change required to reap the benefits of AI.
A recent Guardian survey found that more than 6 million workers in the UK fear being replaced by machines. Although these fears are real, they are founded on only half of the reality of AI’s forecasted impact. The World Economic Forum estimated that by 2025, 85 million jobs will be displaced by AI, but another 97 million new roles will open because of AI. In addition, while automation can sometimes replace human work, more importantly, it is creating more valuable work for humans. David Cremer and Garry Kasparov point out that AI and humans don’t have the same qualities and ability. AI is fast, accurate, and consistently rational, but it lacks the intuitiveness, creativity, and strategy. In this context, AI projects should be viewed from a lens of optimizing human talent, shifting workers away from repetitive, physical, isolated, and dangerous work and toward more variable, strategic, interactive work. In the case of machine health, maintenance and reliability engineers will do less wrench turning and less physical machine inspections, and more targeted repairs and analysis, aided by AI.
Nonetheless, dispelling fear of AI and getting your workforce to engage with it rather than avoid it is essential. The CIO of Accenture rightly stated, “The greater the degree of organizational focus on people helping AI, and AI helping people, the greater the value achieved”. This insight underpins a key question we ask ourselves daily at KCF Technologies, how can we develop and deliver the best AI tools to elevate workers so that they can make a profound impact on business performance?
Citations
Fountaine, Tim. et. al. “Getting AI To Scale.” Harvard Business Review, Winter 2021, pp. 54-60.
Babic, Boris. et. al. “A Better Way To Onboard AI.” Harvard Business Review, Winter 2021, pp. 71-76.
Jesuthasan, Ravin. et. al. “What We Often Get Wrong About Automation.” Harvard Business Review, Winter 2021, pp. 107-109.
De Cremer, David. et. al., “AI Should Augment Human Intelligence, Not Replace It.” Harvard Business Review, Winter 2021, pp. 97-100.