How to Conquer the 5 Most Common Predictive Maintenance Challenges
November 14, 2023 | Machine Health Solutions
In the fast-paced world of manufacturing, optimizing operational efficiency is key to success. Predictive maintenance has emerged as a powerful tool for manufacturers, allowing them to prevent equipment failures before they occur – effectively eliminating unplanned downtime and increasing safety across a variety of critical industries. However, implementing a successful predictive maintenance program comes with its own set of challenges that can be overcome.
Challenge #1: Data Overload and Analysis
Manufacturing operations generate massive amounts of data daily. Analyzing and extracting usable patterns and predicting potential equipment failures from this vast data pool can be a daunting task—even for the most skilled maintenance or reliability professional. When identifying a predictive maintenance solution for your plant, it’s important to consider how this data is used to initiate the right action. In addition to full spectrum and high-definition data, KCF Technologies offers users advanced analytics, machine learning algorithms, and tools to view data in a way anyone can understand.
Challenge #2: Integration with Existing Systems
Integrating predictive maintenance systems with existing manufacturing systems and infrastructure can often be a messy problem to solve. Compatibility issues and the need for smooth data flow between systems can impede successful implementation. The right predictive maintenance system should seamlessly integrate with existing systems, such as Maximo or SAP. The KCF Technologies Platform offers multiple integration types to best integrate with your site’s current system—including the ability to auto generate work orders in external software.
Challenge #3: Cost Constraints
Implementing predictive maintenance can be a significant financial investment. Many manufacturing companies, especially smaller ones, face budget constraints that hinder the adoption of advanced technologies. A machine health provider should offer flexibility in pricing and payment structures to meet the needs of your business. This is why KCF Technologies offers both Capex purchases and SAAS subscription purchases to make predictive maintenance accessible to organizations of various sizes.
Challenge #4: Skill Gap and Training
With the adoption of new technologies comes the need for training experiences to ensure the most effective utilization of predictive maintenance tools. Bridging the knowledge gap and ensuring that the workforce is proficient in utilizing these advanced technologies can be a time-consuming process. Amid labor shortages, this can prove to be an impossible task without the right machine health solutions partner. To successfully transform into a predictive maintenance culture, your machine health partner should be more than simply a vendor. They should be a true extension of your own team. KCF Technologies offers multiple options, dependent on your site’s needs, including our Sentry Services division. In addition, we offer KCF Academy for self-led learning and over 500 courses and counting.
Challenge #5: Scalability
As manufacturing operations expand, the predictive maintenance program needs to scale accordingly to cater to a growing number of machines and equipment. Scalability while maintaining efficiency and accuracy poses a significant challenge. Wireless sensors that can be installed within seconds are key in scalability as well as having a flexible configuration. KCF Technologies Vibration Sensor magnetically mounts, streams data within minutes, and automatically connects to the closest gateway. KCF’s machine health platform is infinitely scalable – unlimited users, unlimited sensors, and unlimited locations.
OPTIMIZE YOUR MACHINE HEALTH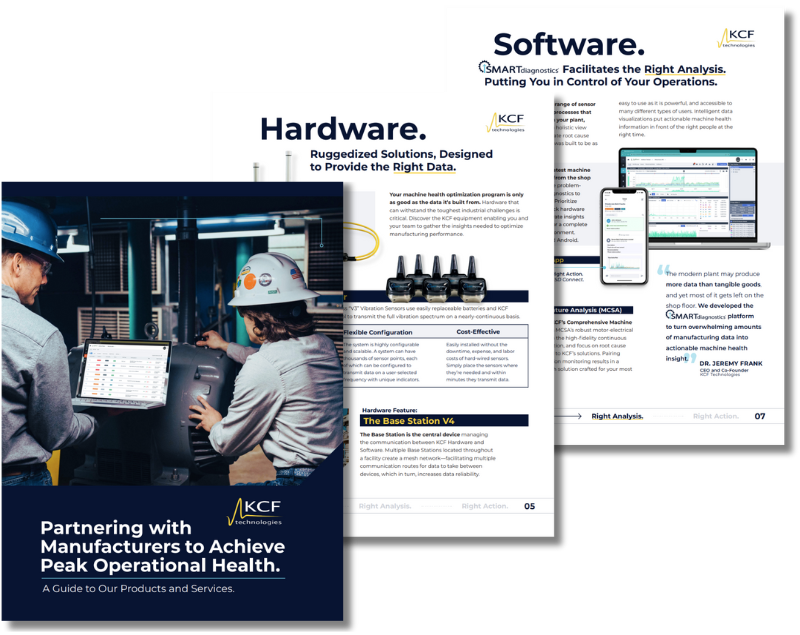
Predictive maintenance is undoubtedly a game-changer for the manufacturing industry. By partnering with innovators like KCF Technologies, manufacturers can effectively combat these challenges and unlock the true potential of predictive maintenance, ensuring smoother operations, reduced downtime, and increased productivity.
You can learn more about our approach to predictive maintenance in the Full Machine Health Guide.